Enhancing Automated Marking : Leveraging Large Language Models and Automated Feedback Generation.
In Progress
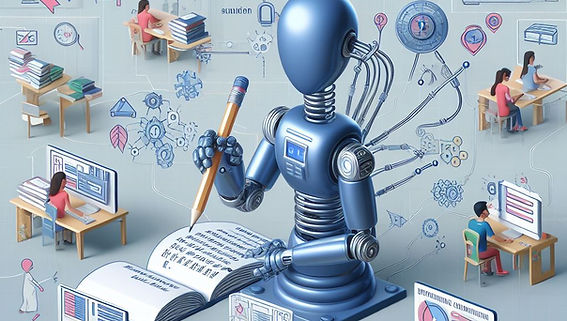
In this research, we explore the potential of Large Language Models (LLMs) to revolutionize the automated marking process in education. By harnessing LLMs' sophisticated understanding of language and instructions following nature, subject knowledge is given with the aim of increasing accuracy in the marking of structured question answers.
Additionally, we will examine the use of grading rubrics to maintain consistency and fairness in the marking process. Through prompt optimization techniques, we seek to enhance accuracy by refining prompts to mark the students answers. Importantly, we will examine the reliability and generalizability of prompts across various subjects, making the optimized prompt applicable to automated student answer marking of various subjects. Finally, detailed feedback generated utilizing the rubric grading scale will provide students with valuable insights to aid their learning journey. Overall, this research aims to leverage cutting-edge language technologies to transform the automated marking landscape.